Artificial intelligence (AI) techniques and methods have attracted considerable attention in the information industry and in society as a whole, due to the large amount of data and the imminent need to transform this data into useful knowledge and practical solutions. However, the effective use of data in some areas is still under development, as is the case in sports. As in most other areas of society, increasing volume of data has been gathered in all kinds of sports, and automated data analysis has become an important and fast developing field.
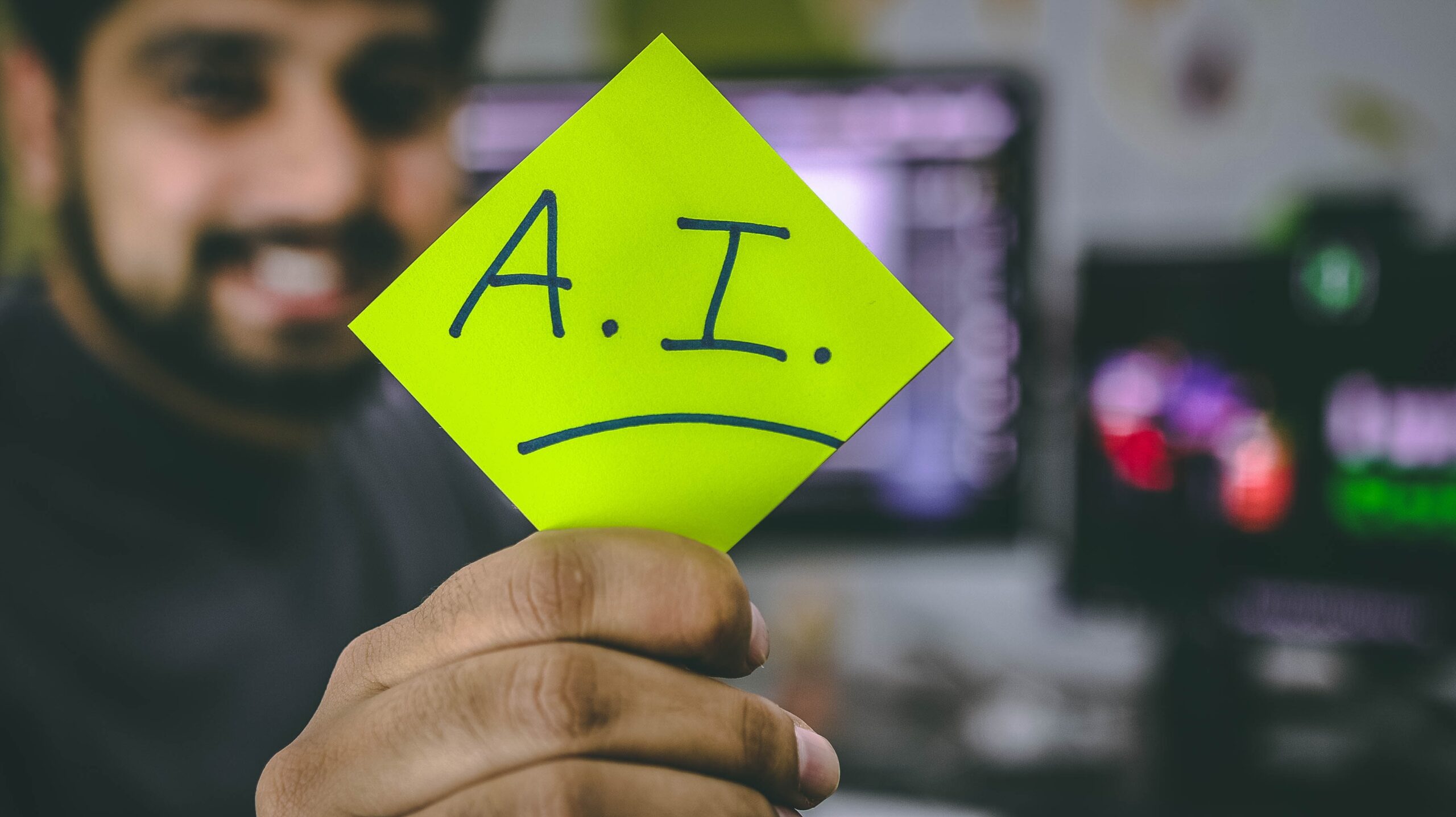
Lapham and Bartlett ’s study was one of the first studies using AI in the analysis of sports performance. In 1995, they demonstrated that the increasing role of computers in the decision-making process, through the use of AI techniques, would be a potentially rewarding future direction for the discipline and already pointed out the artificial neural networks successes in experiments, along with their potential benefits.
The current state of development in the area proposes a promising future with regard to AI use in team sports. Injuries exert an enormous impact on athletes and teams. This is seen especially in professional soccer, with a marked negative impact on team performance and considerable costs of rehabilitation for players. Existing studies provide some preliminary understanding of which factors are mostly associated with injury risk, but scientific systematic evaluation of the potential of statistical models in forecasting injuries is still missing. Some factors raise the risk of a sport injury, but there are also elements that predispose athletes to sports injuries. The biological mechanisms involved in non-contact musculoskeletal soft tissue injuries are poorly understood. Genetic risk factors may be associated with susceptibility to injuries, and may exert marked influence on recovery times. Athletes are complex systems, and depend on internal and external factors to attain and maintain stability of their health and their performance. Organisms, participants or traits within a dynamic system adapt and change when factors within that system change. Scientists routinely predict risk in a variety of dynamic systems such as weather, political forecasting and projecting traffic fatalities. Ideally, the modeling for each forecasting model involves sophisticated statistical assessment, complex/multiple simulation studies, allowances of time-related changes and distinctly large sample sizes. We assumed that baseline characteristics such as strength, balance and flexibility would predict an injurious event, independent of their internally driven change and often independent of external factors.
The typical approach to predict injury risk in the literature has been based on linear regression. While suitable for simple models, linear regression is unable to capture the complex, non-linear interplay between multiple input features. The emergences of sport actions will depend on the task, personal and environmental constraints and the aim of the coach should be to train athletes to adapt their behavior to the constant changing and unpredictable environments.
Since we are attempting to model a very complex system; the human body, across many variables and signals it would be desirable to train artificial intelligence models that exploit non-linear relationships between observable variables, in order to approach complex phenomena such as soft-tissue injuries.
Data science has emerged as a strategical area to exploit knowledge in sports science aiming to fill some gaps left by traditional statistical methods. As a hybrid knowledge area, data science is more than the combination of statistics and computer science as it requires training in how to weave statistical and computational techniques into a larger framework, problem by problem, and to address sports-specific questions . A holistic view of data science requires an understanding of the context of data, appreciating the responsibilities involved in using private and public data, and clearly communicating what a dataset can and cannot tell us about the real world , in our case, in the sports world.
Further evaluation research based on prospective methods is warranted to establish the predictive performance of specific AI techniques and methods. We propose that the use of artificial intelligence may well help in assessing risk and help to predict(prevent) the occurrence of sport injuries.
Author: Georgios Kakavas PT OMT ATC MSc PhDc
Reference: Artificial intelligence: A tool for sports trauma prediction. Kakavas et al. Injury, Volume 51, S63 – S65