The full paper can be found here.
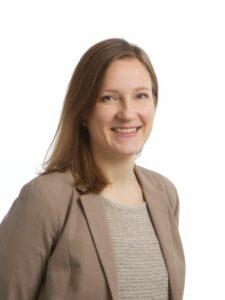
Tell us more about yourself and the author team.
I am a Doctoral Researcher from the Faculty of Sport and Health Sciences, University of Jyväskylä. My research focuses on interpreting and understanding the results gained from physical fitness monitoring systems aimed at school-aged children, with emphasis on the one that we have in Finland (The Move! – monitoring system for physical functional capacity). The author team includes my supervisors, prof. Urho Kujala from the same faculty as myself, Dr Heidi Syväoja and Dr Tuija Tammelin from LIKES Research Centre for Physical Activity and Health and our collaborators Mr Ilkka Rautiainen and Dr Sami Äyrämö from the Faculty of Information technology, University of Jyväskylä.
What is the story behind your study?
As a background, there is a dilemma. Although the physical fitness of school-aged children is commonly assessed, there is still room to improve the given feedback. The 20-m shuttle run test is probably the most used measurement in different physical fitness monitoring systems and part of the Move! system as well. Many previous studies related to the 20-m shuttle run test developed norm and criterion references and discussion about its interpretation. However, especially the individual level accuracy of the criterion references is of concern, and this dilemma could potentially benefit from new methodological approaches.
The origins of this study lead to my participation out of curiosity in a machine learning beginners course organized by the Faculty of Information Technology, University of Jyväskylä. The course started with a lecture and had approximately 100 participants, with me as the only one outside of the Faculty of Information Technology. The course continued with demonstrations where there were only about 20 people left and ended with a seminar where project works were presented with only five persons remaining. On this occasion, I presented the first design of this study but struggled with the analyses. Sami, the lecturer in this course, offered collaboration, and as my supervisors showed the green light, we started to build a manuscript based on this design. In that final seminar, Ilkka became an equal contributor to this manuscript, and this study is also part of his thesis, which focuses on utilizing predictive modelling in health care.
In your own words, what did you find?
Using a machine learning approach, we predicted fitness development during adolescence and identified those individuals to whom exercise interventions could be recommended based on their holistic profile. Up to 20 different characteristics predicted future physical fitness. These variables included a low measured and perceived physical fitness, high markers of adiposity, low markers of physical activity, low academic achievement in school, low life enjoyment, low parental support and low perceived social status at school. Especially the findings that social and psychological factors contribute to fitness trajectories adds to the previous body of research where performance development is typically examined through growth and maturation ignited morphological changes. Therefore, adolescents’ overall physical, psychological, and social status are recommended to be assessed before deciding on interventions based on the 20MSRT score.
What was the main challenge you faced in your study?
It took four years to finish this research. We did not face any unordinary challenges but creating something new with a new research team took its time. In the submission phase, the first journal rejected our manuscript with reviewers’ comments that it was all too abstract and difficult to understand. We had to considerably improve the manuscript and added the framework of precision exercise medicine to further describe the context.
If there is one take-home message from your study, what would that be?
The precision exercise medicine approach holds a potential to prevent and treat diseases with exercise, taking the individual variability into account. This study provides an example with attached scripts and functions on using machine learning for similar purposes.