In “past, present, future”, we ask clinical or academic experts to reflect on selected Sports & Exercise Medicine topics. Today Ian Shrier on Research Methods & Statistics in Sports & Exercise Medicine
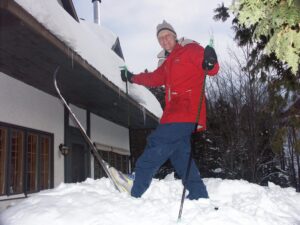
Tell us more about yourself
After finishing training in medicine, I spent 3 years practising sport and exercise medicine and emergency medicine. To fulfil my creative needs, I started a PhD in basic science physiology, followed by a post-doc in Epidemiology and clinical research. After a few more years, I started using injury data and found that this field’s methods often failed to follow standard principles. For example, methods didn’t account for repeated measures on participants and often lacked appropriate calculations for confidence intervals. I was very fortunate to find a statistician who was willing to become “invested” in improving the application of appropriate methods. I have learned a great deal through our collaborations over the last 20 years. The most important lesson I have assimilated is not to overestimate the value of my own experiences and always seek out appropriate expertise to answer research questions.
What was hip and happening 10 years ago?
Surveillance databases in sport and exercise medicine started to become available about 10-15 years ago. That was hip because sports medicine clinicians and researchers could track large groups of athletes over time. There was great promise and excitement, and it was a great first step. As the databases from the last 10 years have matured, investigators can now address important questions that were simply not possible to answer before. At the same time, there were important limitations. Our field simply did not keep up with the methodological advances being made, and despite good intentions, some investigators proposed methods without fully understanding the mathematical principles of the methods. This led to some sub-optimal practices becoming widely used.
What are we doing now?
In recent years, there has been a push towards using modern methods to estimate causal effects rather than just predictors of outcomes (mix of causal and non-causal effects). This is a positive move. However, this comes with a caution. Causal effects provide answers to only some of the important clinical questions. Some journals and reviewers do not appear to understand that some non-causal questions are also very important. I think this is unfortunate. Non-causal questions may require simple or advanced methods depending upon the question being asked. The pendulum swings back and forth. So we must always keep in mind that we start with an important question and then choose methods that will answer that question. My favourite sport growing up was tennis. I think we are still trying to figure out where the “sweet spot” of the racquet is regarding the optimal methods for different types of causal and non-causal questions.
Where do you think we will be 10 years from now?
I am hopeful that we can continue to improve methods used in both consensus statements and original research. I recently published an article in BJSM (“Consensus statements that fail to recognize dissent are flawed by design: a narrative review with 10 suggested improvements”) explaining why I think the current process to create consensus statements is seriously flawed even though they follow “recommended” practices. My paper highlighted some concrete examples where recommendations were sub-optimal and ideas on how to improve the process. Most importantly, organizers need to encourage dissent in both actions and words. This requires inviting collaborators with a full range of scientific opinions and methodological expertise. Organizers also need to report the level of agreement for each recommendation and the major dissenting opinions. For original research papers, our field would improve greatly if every paper with a quantitative analysis included a statistician as a co-author responsible for (1) the data analysis and (2) ensuring that the results are properly reported and interpreted. There is an old joke that the definition of an epidemiologist is someone who everyone calls a statistician, except the statisticians. Alternatively, in the words of Andrew Vickers, PhD, “A mistake in the operating room can threaten the life of one patient; a mistake in statistical analysis or interpretation can lead to hundreds of early deaths. So it is perhaps odd that, while we allow a doctor to conduct surgery only after years of training, we give SPSS to almost anyone”. [Vickers A. Interpreting data from randomized trials: the Scandinavian prostatectomy study illustrates two common errors. Nat Clin Pract Urol, 2005]”