The number of studies assessing the diagnostic accuracy of tests is growing rapidly, but many studies fail to impact on practice due to five fundamental flaws in their methods.
Jack O’Sullivan
Diagnostic accuracy studies aim to determine how good a new test is at diagnosing a disease compared with a current test. To do this, investigators subject study patients to both the new test (also called the ‘index test’) and the current test (also referred to as the ‘reference standard’). The number of patients that test positive to both tests is then determined (‘True Positives’), as are the number that tests negative to both (‘True Negatives’).
Just to make a little bit more complicated, the patients that test positive to the index but negative to a reference standard (‘False Positives’) and those that test negative to the index test but positive to a reference standard (‘False Negatives’) are also generated. These results can then be used to calculate, amongst other metrics, a sensitivity and specificity.
Over the last 40 years, more than 6,500 diagnostic accuracy studies have been published; an increase of more than 2000% (Figure 1).
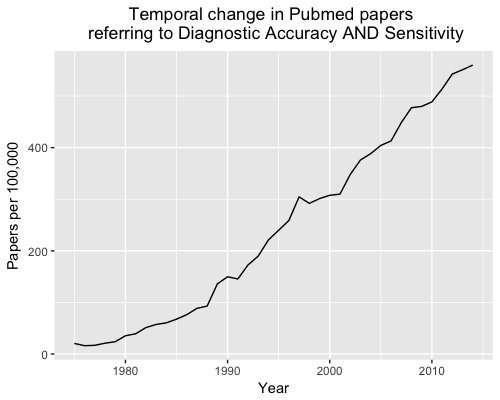
However, there is still considerable waste in biomedical research and so little of the increasing volume of research translates into practice. Here are five reasons why diagnostic accuracy studies often fail to impact on practice.
1. Diagnostic Accuracy Studies don’t capture the whole diagnostic pathway
Diagnostic accuracy studies examine only one aspect of a patient’s journey – diagnosis. These studies don’t follow patients through their journey to treatment and/or further testing. Diagnostic accuracy studies, therefore, don’t provide data on important outcomes such as mortality, quality of life or morbidity that matter to patients.
Diagnostic Randomised Controlled Trials (D-RCTs) study the entire patient pathway, from diagnosis to treatment: randomising patients to either receiving or not receiving a test and then follow up outcomes such as mortality and morbidity. In this way, D-RCT studies account for ‘the complexity that originates from physician decisions, further interventions, and patient perspectives toward test results’.
2. Inappropriate Gold (Reference) Standards
Diagnostic accuracy studies require an appropriate reference standard (also known as the gold standard test) to compare with the new test. If the reference test does not classify patients as diseased or not diseased, then the accuracy of a new test will not be valid. For example, If investigators choose a less accurate test (such as urine dipstick) as the reference test, rather than urine culture (more accurate test), the accuracy results of the new test will be biased.
3., Lack of blinding
Study investigators should be blinded to the result of the index test when assessing the result of the reference standard (and vice versa). Knowledge of either of these results can bias interpretation and thus affect results. This is particularly relevant for tests that have a subjective interpretation. Consider a CT scan being used being used as the reference standard in a study. The knowledge that a new index test classified a patient as diseased could influence a radiologist’s subjective assessment of the CT scan.
4. Non-random or non-consecutive recruitment of patients
Random or consecutive recruitment of patients suspected, but not known to have, the target disease helps ensure results of diagnostic accuracy studies are relevant and applicable to real-world clinical practice.
Clinicians often employ tests when diagnostic uncertainty exists;. If diagnostic accuracy studies recruit patients that have a high and/or low likelihood of actually having the disease, the study results may make the test appear more or less accurate than in the real world. Consider a study aiming to determine the accuracy of a new test to diagnose heart failure. If the study recruits confirmed heart failure patients directly from a heart failure clinic, the new test may appear more accurate than if it recruited patients presenting to primary care with heart failure symptoms.
5. Verification bias
Verification bias refers to a measurement bias in which not all patients receive the reference standard (subtype: partial verification bias) or patients don’t all receive the same type of reference standard (subtype: differential verification bias). This may be done for ethical or feasibility issues, nevertheless, it risks misclassifying patients; particularly false negatives. Consider the example of a study determining the accuracy of faecal occult blood tests (FOBT) to diagnose colorectal cancer (CRC). It might seem reasonable for feasibility and ethical reasons for study investigators to only subject patients with a positive FOBT to the reference standard test (colonoscopy), but this means all patients that test negative to FOBT will be considered ‘true negatives’, misclassifying false negatives: those that would be diagnosed with CRC on colonoscopy.
Diagnostic tests have a crucial role to play in improving patient outcomes. Awareness of the above rules will help ensure that the investment in more diagnostic accuracy studies translates into clinical practice.
Jack O’Sullivan, Editorial Registrar BMJ EBM, Dr & DPhil Candidate at the University of Oxford
Conflict of interest: My DPhil (PhD) is funded by the Clarendon Fund, University of Oxford. I also receive income from Oxford University Hospitals for clinical work and hold grants from the National Institute for Health Research and the Primary Care Research Trust. I have no conflicts of interests.