By Natasha Karp
To improve the reproducibility, we need to change the way we design our in vivo experiments
To understand the effect of a treatment, our experiments simplify a complex world by generating control and treatment groups allowing us to isolate cause and effect. This generates an inference or testing space, which arises from the population sampled. From these studies, we then draw conclusions and generalise the results to a broader population. The level of complexity in biology is such that we do have to make hard choices when we design experiments. We cannot explore the impact of all sources of variation on the treatment effect, rather to progress our scientific understanding we need to generate “doable problems” [1] allowing us to incrementally unravel the biological story. The danger presented by too narrow a testing space is that external validity will be low, and results will be so context-dependant that they are hard to reproduce or generalise. The value of many animal studies could be improved by giving further consideration to these effects
The reality of our testing space
The primary strategy used to manage potential sources of variation within in vivo research is strict standardisation. We typically standardise the animals (e.g. inbred animals of one sex), the environment (e.g. standard housing and husbandry conditions), the testing procedure and time (e.g. conducting the experiment in one batch in time). The drivers behind this are two-fold. Firstly, to manage potential confounders to ensure that the only difference between the control and treated groups is the treatment. The second driver arises from the objective to reduce the variation in the data with the argument that this will result in fewer animals being needed to detect a defined effect size of interest. This argument frequently dominates our discussion when selecting the right experimental design because of the historic interpretation of “Reduction” from the 3R (Replace, Reduce and Refine) ethical framework where we focused on minimizing the number of animals within a single experiment. If we relate this to a clinical study, this would be equivalent to testing a treatment on 30-year-old identical twin brothers who all live in the same town, followed the same career path and now live a life with the same monotonous diet and exercise routine [2]. Reflecting on the scenario raises several questions. Why would this observed effect in such a standardised situation be representative of a population? Why do we expect an effect observed in this standardised situation, with artificially low variation, to translate to a meaningful effect once normal biological variation is present?
In reality, for most scenarios, the treatment effect is context dependent.
Living organisms are highly responsive to the environment with phenotypic changes with both long (e.g. development) and short-term (e.g. acclimation) duration [3]. This phenotypic plasticity is an essential component for survival and is an evolutionary adaption methodology to ensure optimal fit. Without phenotypic plasticity there would be no treatment effect. For some treatments, the effect could be robust and only depend on the nature, duration and intensity of the treatment. It is more likely that the effect of the treatment also depends on the animal’s current phenotype which arises from its history, genotype and the experimental context. The initial reaction to this problem was a call to standardise further and report the context [4–6]. However, research has shown that this leads you down a rabbit hole. Animals are so responsive that you cannot standardise sufficiently to reproduce the context [7, 8]. It raises the question of what is the value of identifying effects that are idiosyncratic to a unique environment and only significant when bench marked against technical variation as biological variation has been minimised.
We need a paradigm shift in our designs, reporting and conclusions
Eleven experts, working on this arena, have proposed a paradigm shift in how we conduct our experiments to improve reproducibility [9]. They propose we should embrace variation, but not randomly; rather, they recommend systematic heterogenization by utilising random block designs (Fig. 1). With a block design, the conditions (environment or aspects of the animal) are standardised within a block; however, between blocks conditions can vary. With this type of design, we can estimate the average effect across the blocks but also explore the consistency of this response.
Such a design has recently been demonstrated for tumour studies [10] to deliver a confidence estimate of the treatment effect for a defined environment by splitting the experiment into a series of small independent mini-experiments. The research showed that the design reduced the need to replicate the experiment, which can reduce the total number of animals used. There are questions still to answer, such as what factors to heterogenise on.
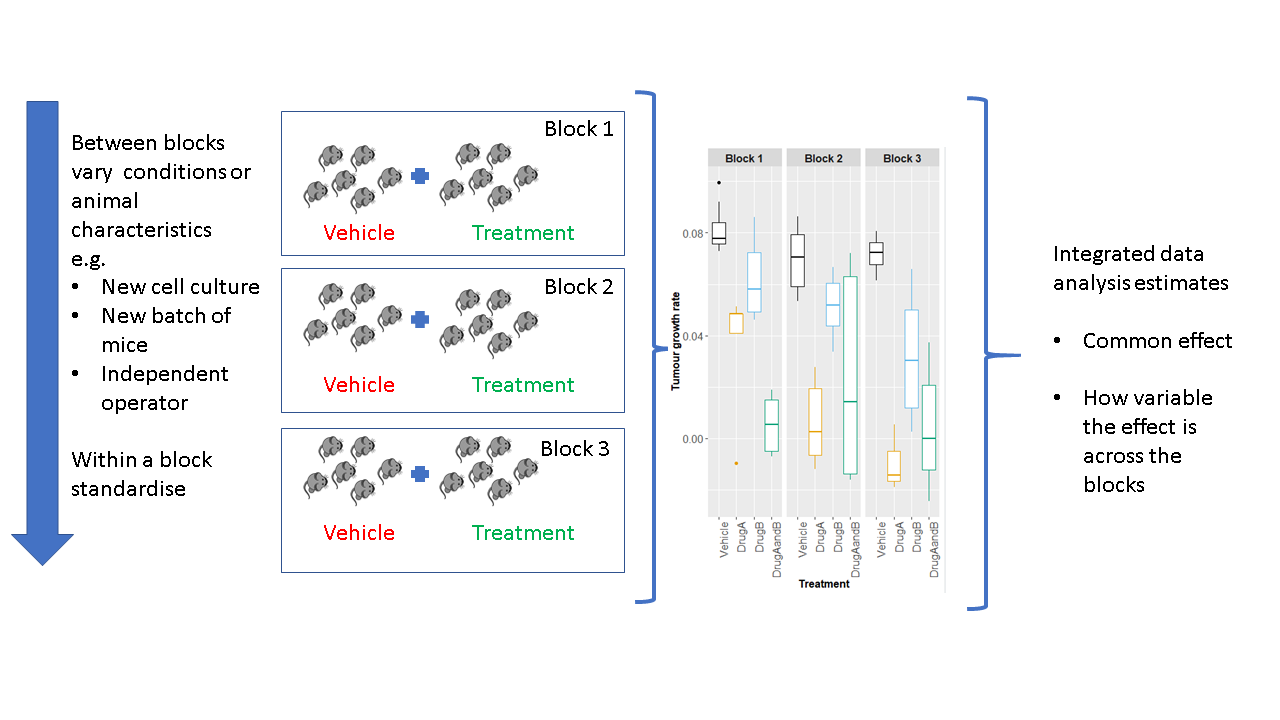
Meeting our ethical obligations requires us to change practice
Our current practice with highly standardised experiments, tests a narrow inference space, which leads to a significant risk that the estimated effects will be over confident and idiosyncratic and will not be reproducible. We need a mindset change, to focus on maximizing the amount of knowledge gain per animal and/or per study rather than minimizing the number of animals per study. The focus on the sample size within a single experiment is resulting in poor quality experiments that will lead to more animal use in the long run. The need to change our interpretation is reflected in the contemporary definition of Reduction by the National Centre for the Replacement, Refinement and Reduction of animals in Research (NC3Rs), which now includes “… experiments that are robust, reproducible and truly add to the knowledge base”[11]. The first steps on this journey is to acknowledge the reality of the testing space we are working within. The second step is to embrace block designs to gain more confidence in the observed treatment effects. These together will enable us to understand the limitations of our testing space and actively improve the inference space we are working within.
References:
- Gompers, A., Dec 10 Dec 10 Three Years In:“Sex as a Biological Variable” Policy in Practice-and an Invitation to Collaborate.
- Garner, J.P., et al., Introducing Therioepistemology: the study of how knowledge is gained from animal research. 2017. 46(4): p. 103.
- Voelkl, B. and H.J.T.i.p.s. Würbel, Reproducibility crisis: are we ignoring reaction norms? 2016. 37(7): p. 509-510.
- Omary, M.B., et al., Not all mice are the same: standardization of animal research data presentation. 2016. 65(6): p. 894-895.
- Beynen, A., M. Festing, and M. van Montford, Design of animal experiments. Principles of Laboratory Animal Science, 2nd edn. van Zutphen LFM, Baumans V, Beynen AC, eds. 2003, Amsterdam: Elsevier.
- Mering, S., E. Kaliste-Korhonen, and T.J.L.A. Nevalainen, Estimates of appropriate number of rats: interaction with housing environment. 2001. 35(1): p. 80-90.
- Crabbe, J.C., D. Wahlsten, and B.C. Dudek, Genetics of mouse behavior: interactions with laboratory environment. Science, 1999. 284(5420): p. 1670-1672.
- Karp, N.A., et al., Impact of temporal variation on design and analysis of mouse knockout phenotyping studies. PloS one, 2014. 9(10): p. e111239.
- Voelkl, B., Altman, N.S., Forsman, A. et al. Reproducibility of animal research in light of biological variation. Nat Rev Neurosci (2020).
- Karp, N.A., et al., A multi-batch design to deliver robust estimates of efficacy and reduce animal use–a syngeneic tumour case study. 2020. 10(1): p. 1-10.
- NC3Rs. The 3Rs. 2018 [cited 2018 16th January 2018].
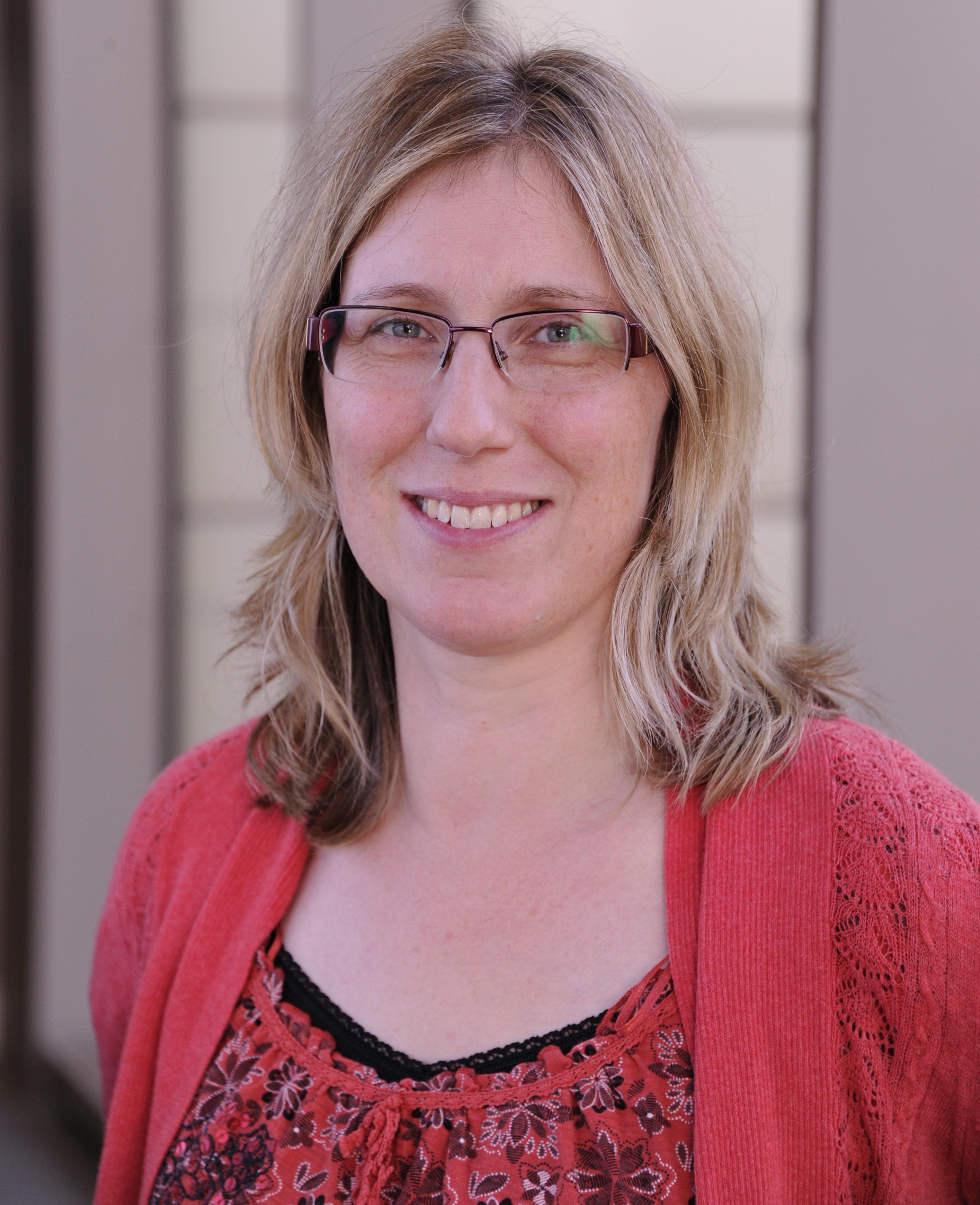
Natasha A Karp, Data Sciences & Quantitative Biology, Discovery Sciences, R&D, AstraZeneca, Cambridge, UK
Conflict of interest: Natasha Karp, is an employee of AstraZeneca and holds shares in this pharmaceutical company. However, as a blog on experimental methodology, there are no conflicts of interest with the subject matter or materials discussed to declare.